How to drive accuracy and save time in KYB and credit underwriting
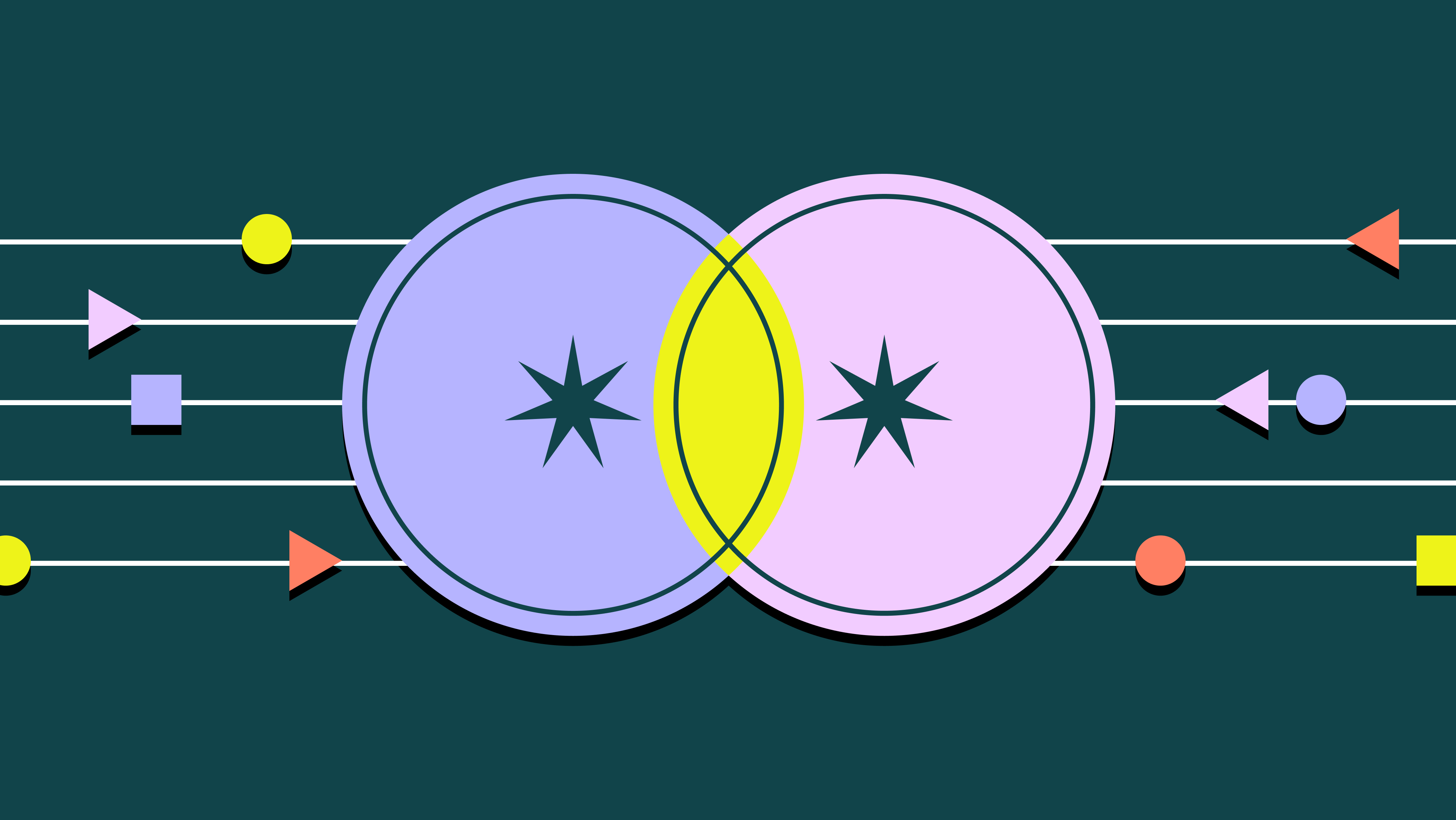
Some businesses have clear vision of what their credit offering should look like. But sometimes, when they go about actually building it, the smallest building blocks can become huge stumbling blocks.
Building and embedding credit-based products can be operationally challenging. Despite an abundance of tools and platforms available, things are not always straightforward and obstacles abound.
In the B2B arena, businesses validate their customers’ identities and assess their financial resilience. They rely on payment histories, but they’ll also tap into a range of 3rd party data sources as well. Sounds simple, right?
In this article I’ll share insights on challenges our partners met while introducing credit-based products, and how we were able to overcome them.
Extending credit can be daunting
It seems straightforward: connect to the database, find the company you’re looking for, fetch the data, analyze and assess it and make decisions accordingly. But in reality, it doesn’t always work like that.
KYB compliance and credit underwriting are complex, and involve cross checking data across various databases. At the root of the issue are strings - linear sequences of characters (words, or other data.) Your customers provide you with strings like company name, company ID, company address, and you need to locate these in various databases to verify the existence of the entity, and to retrieve data pertaining to it.
This is achieved through policies. These policies are built from a series of “If-Then” rules. For example, a rule that compares a provided company name with a company name in a datasource. A minor task that can be easily automated, right?
But should that white space be there? Is it “LTD” or “ltd” or “Ltd.”? Anyone familiar with KYB and credit underwriting will recognize these “issues” which can make simple data queries a pain.
When a simple task like writing rules to compare two strings is done wrong, it can adversely impact your business goals. It can impede automation and can lead to wasted resources and costly mistakes.
Removing obstacles to drive efficiency: Automation
One of our partners pulls reports from Creditsafe by registration number and then verifies the company based on the name. Name verification queries were failing due to the many variants of the appendix BV, B.V., b.v. etc. - a Dutch indication for private limited companies. The different variants were throwing off the query and the manual review team was overwhelmed.
The solution was simple: using a fuzzy match comparison would ignore case insensitivities and would prevent punctuation from throwing the system off.
Now our partner embeds our custom built Match Ratio comparison directly into their policy.
Removing obstacles to drive efficiency: Accuracy
Another partner wanted to consider only businesses that provided a Savings account and knock out any that didn’t as part of their underwriting process. But some borrowers supplied internally branded account types (i.e. Business Select Savings.) Their policies were not recognizing all Savings account types and many valid requests were declined.
The solution was to embed our custom Partial Ratio comparison to look for the short string “Savings” within the provided string (i.e. myBusiness Select Savings.)
Now our partner's rule is more concise - invalid applications are being declined, and credit underwriting policies are only executed on qualified applicants.
Both cases demonstrate the need for a refined mechanism to optimize KYB and underwriting to save time and resources. But there are many string types to compare, so what works best for each scenario and how many resources can a business building credit-based products allot for such cases?
How Fuzzy Wuzzy? (or which comparison to choose)
When a borrower provides a company name, ID and address, there are often discrepancies with various data sources. Fuzzy matching helps overcome these issues by comparing two strings and providing a score to attests to the similarities. Fuzzy matching is commonly used when accessing 3rd party data sources, but there are nuances.
Match Ratio
So the March ratio is particularly useful when looking for a strict comparison.
Partial Ratio
Partial Ratio takes the shortest string and compares it with all the substrings of the same length. It is designed to locate String A within String B, or vice versa. If we compare the strings “7632” and “07632-2505” we get the following scores:
- Match Ratio: 50
- Partial Ratio: 100
Partial Ratio is advantageous when searching for a single string’s presence within a longer string, which is often the case with knock-out rules.
Token Sort Ratio
In Token Sort Ratio, words are tokenized, sorted alphabetically and joined together before they are compared. The order of the words in the strings is irrelevant. Like Match Ratio, varying string lengths will result in a lower score.
If we compare “united states v. nixon” and “Nixon v. United States” we get the following scores:
- Match Ratio: 59
- Partial Ratio: 74
- Token Sort Ratio: 100
The Token Sort Ration is useful when seeking similarity in strings that are of similar length, and where order is of less relevance.
Token Set Ratio
When two strings vary in length, the Token Set Ratio will come in handy. Instead of just tokenizing, sorting alphabetically, and then joining the tokens back together, Token Set Ratio takes out the common words and then compares the new strings.
When comparing "125 Broad Ave, Building C-Unit 18" and “125 Broad Ave Ste 18" we want to find a mechanism that can isolate key words.
How do the various match features score the similarity between these two strings?
- Match Ratio: 69
- Partial Ratio: 70
- Token Sort Ratio: 69
- Token Set Ratio: 89
Token Set Ratio is the clear winner, as it is insensitive to differences in string lengths, as opposed to the Token Sort Ratio.
So, what is the best fuzzy match to use when trying to verify a business’ identity?
Drum roll please… (Here are the insights!)
The above analysis adheres to dry logic. But to reach wider conclusions, we checked the different mechanisms against actual data. Our data analysts funneled real lists of company names, street addresses and zip codes into our algorithms and compared them against those registered in data sources. We aggregated results to conclude which comparison mechanism delivered the most accurate scores.
These were the results:
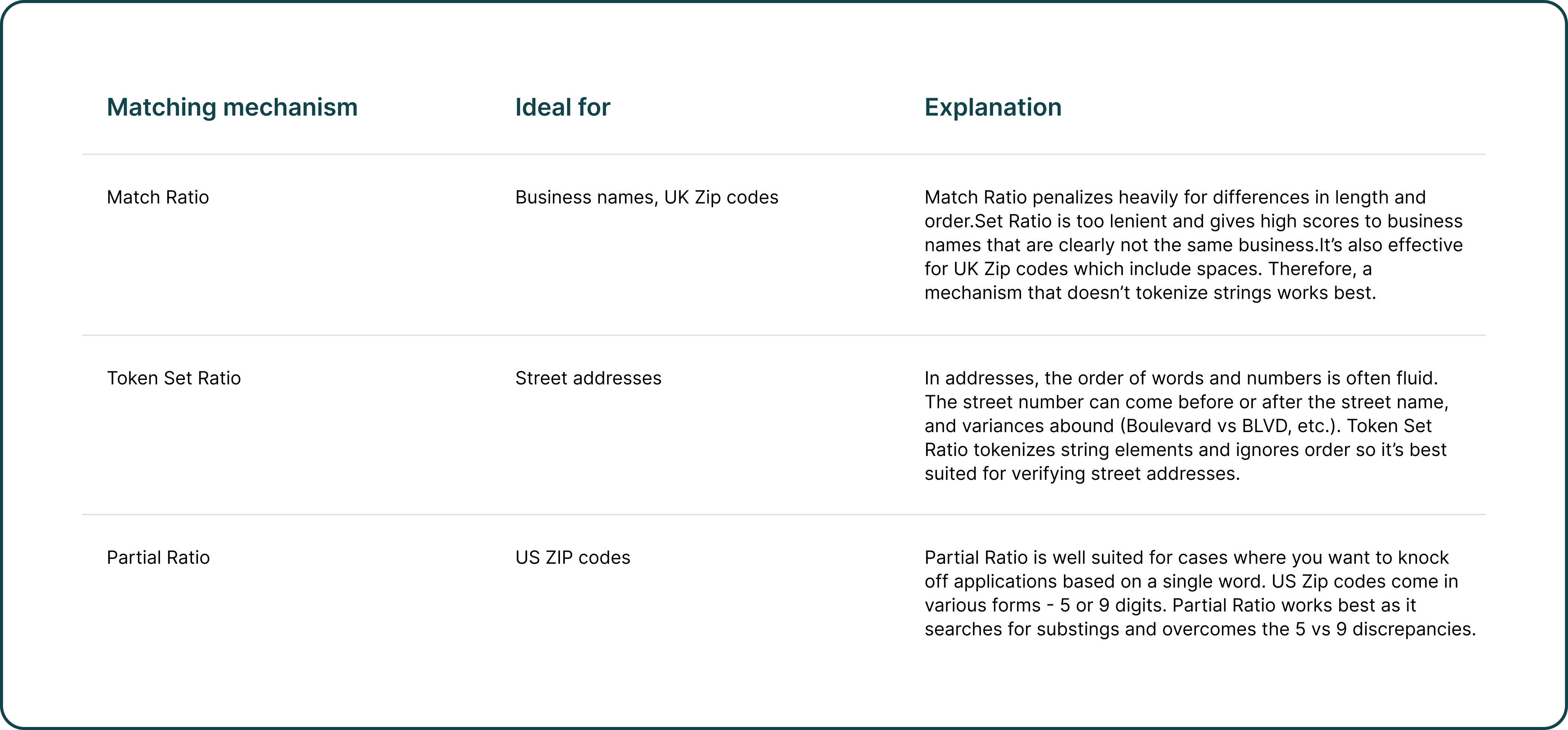
As you may notice, the Token Sort Ratio wasn’t a good indicator for any of our fields. It is too sensitive to string length. It can be replaced by the Match Ratio when the order of both strings is identical and by the Token Set Ratio when the order of both strings differs.
Let your platform do the heavy lifting
Underwriting is a critical part of any credit offering. Businesses need to ace it - to retrieve, aggregate and analyze enough data to reach a clear decision within seconds. Imagine how sidetracked your teams can get when things go wrong and your queries don’t deliver, often for reasons as trivial as an extra space?
The illustrated examples show how damaging a process that falls short can be - even with something as simple as executing a fuzzy match can be: inundating manual review teams, declining applications based on faulty queries, or extending credit based on wrong data.
A resilient KYB and underwriting platform must provide its users with access to a full range of comparison features, as well as functions to enable calculations. Sometimes the data retrieved is raw, and being able to manipulate it is vital. It should also embed insights from domain experts, so that your team can operate smoothly.